When an older timestamp is inserted into a time series, the chunk of memory corresponding to the new sample’s time frame will potentially have to be retrieved from the main memory (you can read more about these chunks here). When this chunk is a compressed chunk, it will also have to be decoded before we can insert/update to it. These are memory-intensive—and in the case of decoding, compute-intensive—operations that will influence the overall achievable ingestion rate.
Ingest performance is critical for us, which pushed us to assess and be transparent about the impact of the out-of-order backfilled ratio on our overall high-performance TSDB.
To do so, we created a Go benchmark client that enabled us to control key factors that dictate overall system performance, like the out-of-order ratio, the compression of the series, the number of concurrent clients used, and command pipelining. For the full benchmark-driver configuration details and parameters, please refer to this GitHub link.
Furthermore, all benchmark variations were run on Amazon Web Services instances, provisioned through our benchmark-testing infrastructure. Both the benchmarking client and database servers were running on separate c5.9xlarge instances. The tests were executed on a single-shard setup, with RedisTimeSeries version 1.4.
Below you can see the correlation between achievable ops/sec and out-of-order ratio for both compressed and uncompressed chunks.
Compressed chunks out-of-order/backfilled impact analysis
With compressed chunks, given that a single out-of-order datapoint implies the full decompression from double delta of the entire chunk, you should expect higher overheads in out-of-order writes.
As a rule of thumb, to increase out-of-order compressed performance, reduce the chunk size as much as possible. Smaller chunks imply less computation on double-delta decompression and thus less overall impact, with the drawback of smaller compression ratio.
The graphs and tables below make these key points:
If the database receives 1% of out-of-order samples with our current default chunk size in bytes (4096) the overall impact on the ingestion rate should be 10%.
At larger out-of-order percentages, like 5%, 10%, or even 25%, the overall impact should be between 35% to 75% fewer ops/sec. At this level of out-of-order percentages, you should really consider reducing the chunk size.
We've observed a maximum 95% drop in the achievable ops/sec even at 99% out-of-order ingestion. (Again, reducing the chunk size can cut the impact in half.)
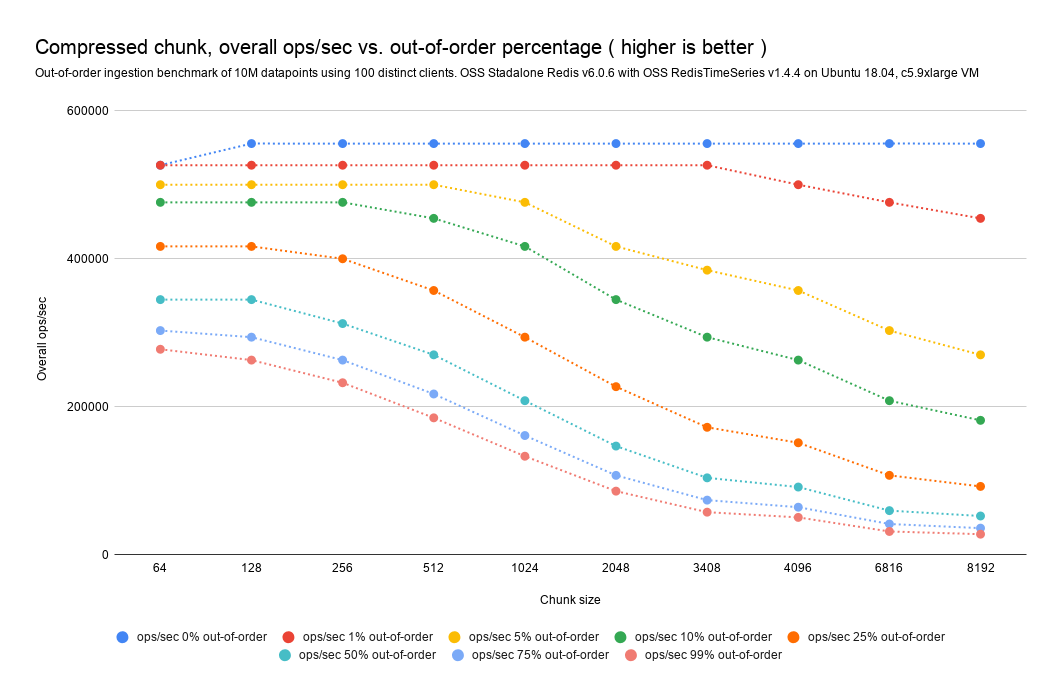
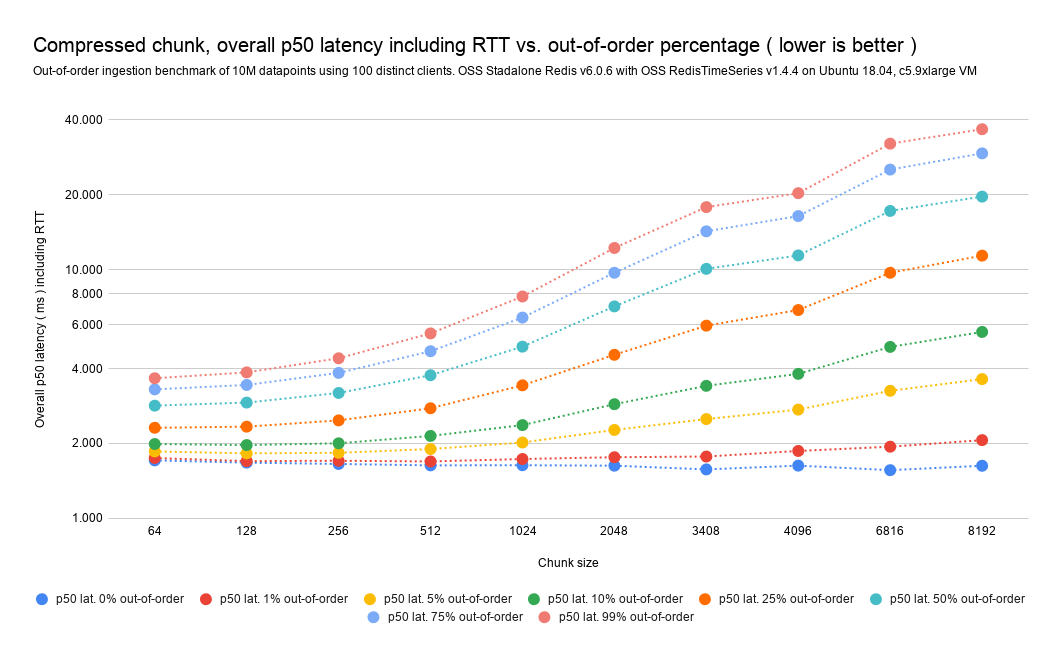
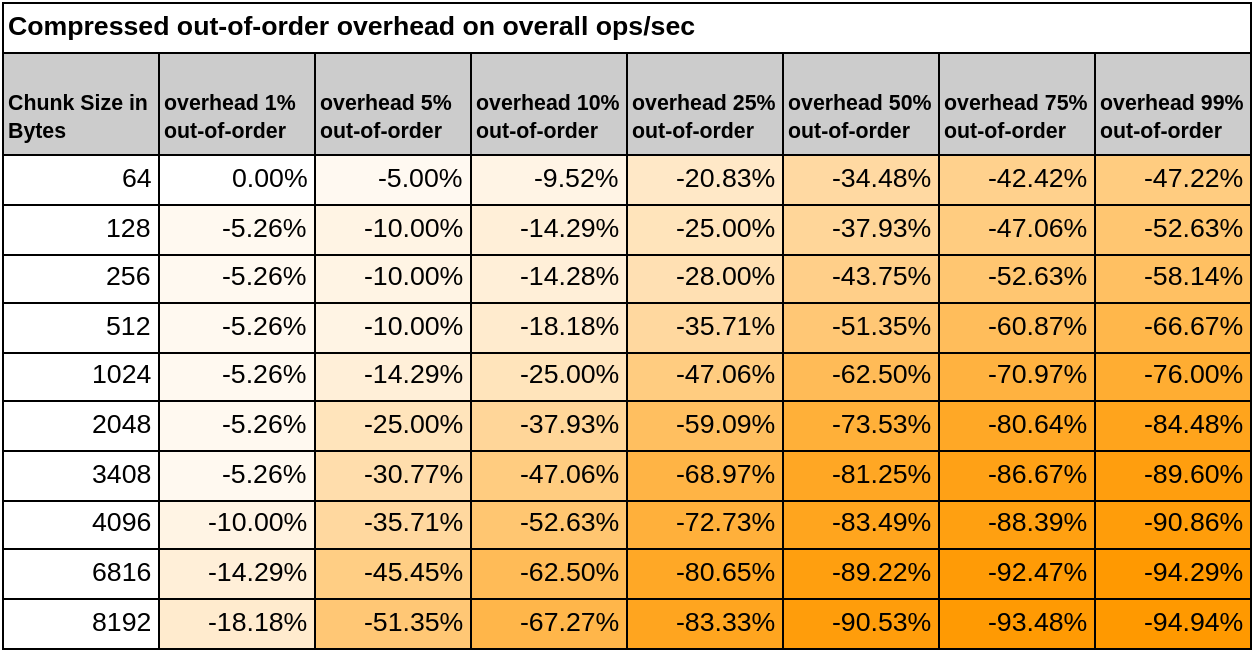
Uncompressed chunks out-of-order/backfilled impact analysis
As visible on the charts and tables below, the chunk size does not affect the overall out-of-order impact on ingestion (meaning that if I have a chunk size of 256 bytes and a chunk size of 4096 bytes, the expected impact that out-of-order ingestion is the same—as it should be). Apart from that, we can observe the following key take-aways:
If the database receives 1% of out-of-order samples, the overall impact in ingestion rate should be low or even unmeasurable.
At higher out-of-order percentages, like 5%, 10%, or even 25%, the overall impact should be 5% to 19% fewer ops/sec.
We've observed a maximum 45% drop in the achievable ops/sec, even at 99% out-of-order ingestion.
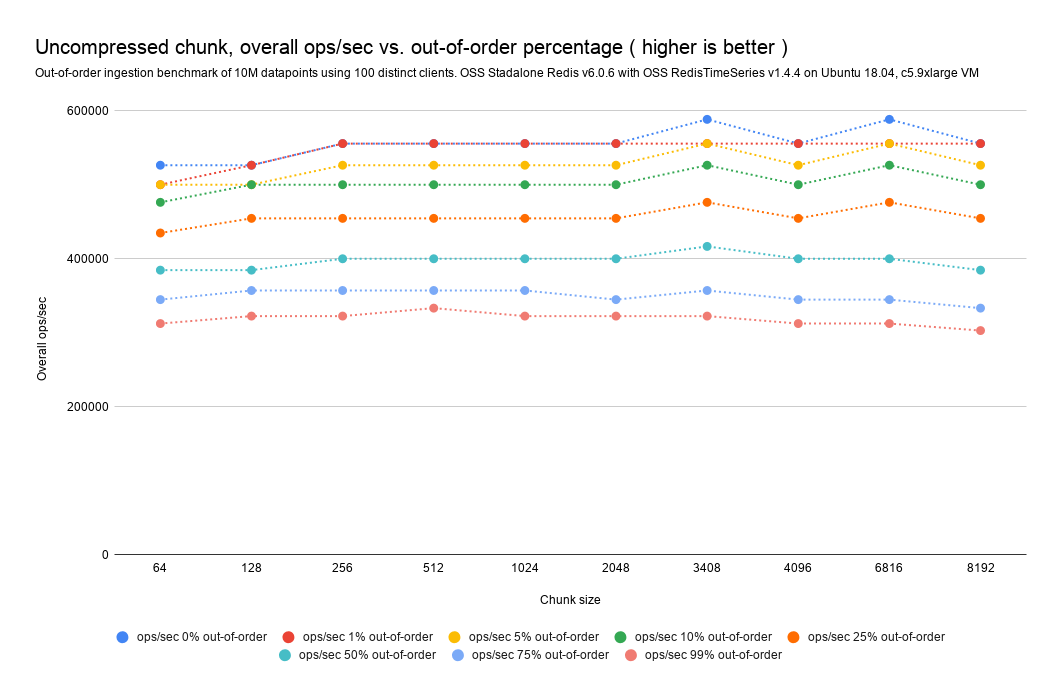
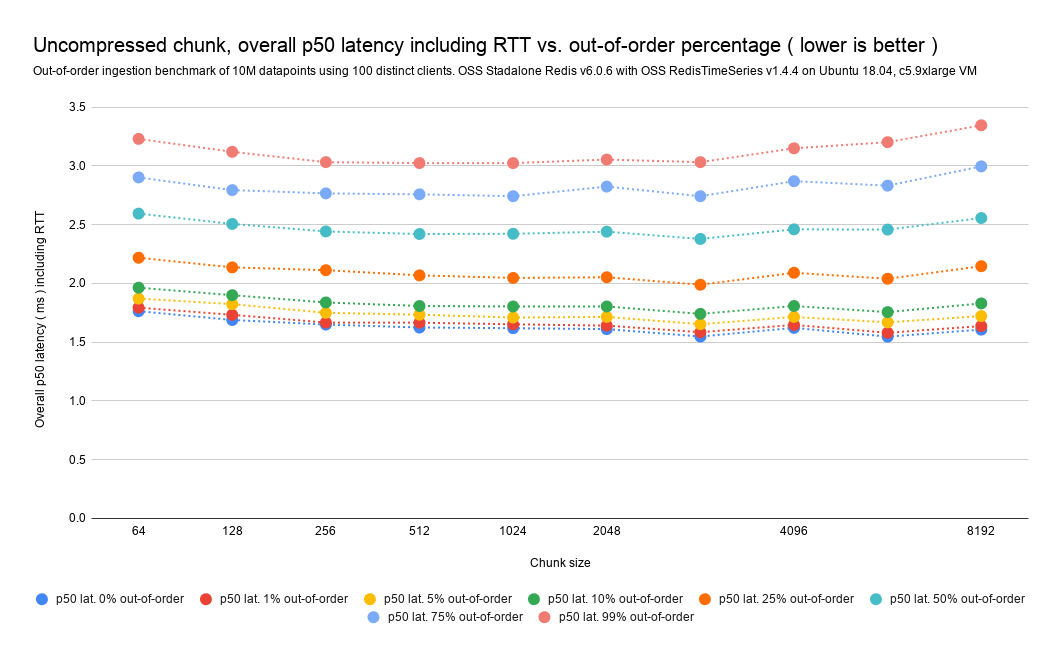
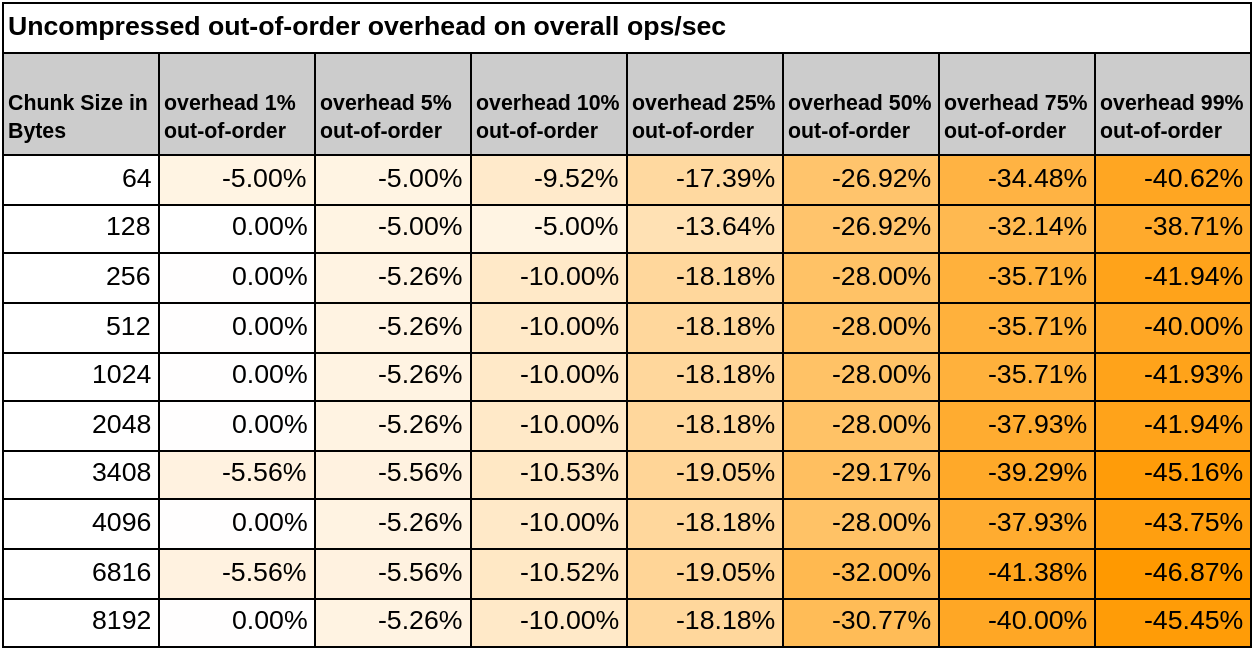